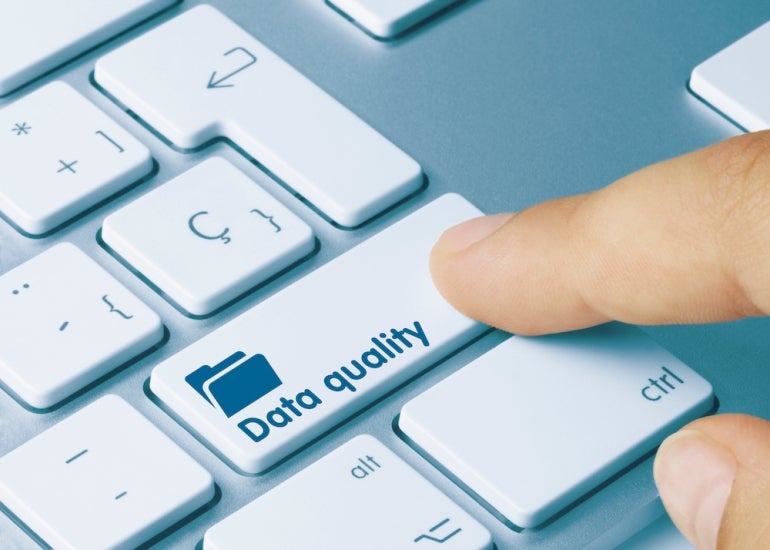
From cost reduction to improved efficiency, upholding data quality improves the accuracy of analytics and enhances business decision-making capabilities. However, simply having a data quality management strategy might not be enough for businesses that want to scale their data operations.
SEE: Hiring kit: Automation specialist (TechRepublic Premium)
Manual data quality management approaches in particular can sabotage data quality, especially with the potential for data entry and other human errors. Beyond this possible problem, manual data quality management also requires hands-on tactical work from data professionals who could otherwise work on more strategic business tasks. The simple answer to both of these problems? Find ways to automate your data quality processes.
Why data quality processes should be automated
Processes such as manual data entry are tedious enough to make it easy to introduce human error. Errors ranging from a simple undetected typo to an entry filled in the wrong field or missed entirely can significantly impact data quality.
SEE: Best practices to improve data quality (TechRepublic)
The solution to this frequent error lies in automating data quality processes, thus accelerating and raising both the efficiency and the accuracy of data quality management. Since automation does not suffer fatigue or lapses in concentration, it is not susceptible to the same data entry errors that humans struggle with. The right configuration of automated data quality processes — using the correct rules and integrations — ensures that data quality automation will improve overall data quality.
Steps to automate data quality processes
Set data quality standards
A strategy to automate data quality begins with understanding and establishing the importance of data quality to the organization. Data quality indicators to study include accuracy, relevance, completeness, timeliness and consistency.
However, the way you approach these indicators is dependent on the goals of the organization and the nature of its data. An organization could, for example, create software-based rules founded on its business requirements, which govern operations and analytics.
Implement strict controls over incoming data
Using third-party data sources can lead to working with large volumes of bad data. Ingesting such data into an organization’s pipelines may be expensive to correct in terms of time and cost. To avoid this, organizations should consider implementing strict control over all incoming data to verify data quality earlier in the process. Nonetheless, verifying data quality from these sources can prove to be a challenge.
Automation can simplify these data quality checks for third-party data. Consider setting up automated data quality alerts that are capable of flagging anomalies, incomplete entries and unusual data formats. With this approach to data quality automation, companies can proactively handle data issues before they enter their pipeline.
Define issue remediation based on organizational use cases
Once bad data has been discovered, issue remediation comes into play to ensure bad data is correctly dealt with. To automate issue remediation, it is necessary to first determine what can be automated and what requires the oversight of a data steward. This helps to clarify who or what should solve each data issue, what can be done in specific use cases and when issues should be escalated to a trained data professional.
Select the right automation tools for your business needs
Automated tools save time, improve efficacy when flagging inaccuracies in data and ensure that data meets required quality metrics. Choosing the correct automation tools, however, requires an understanding of data quality tool limitations. Data quality tools cannot fix data that is completely broken; they cannot cover the shortcomings of an organization’s data framework.
SEE: How do I become a data steward? (TechRepublic)
To derive the greatest value from automation, organizations should carry out a thorough analysis of the right tools and platforms based on their business needs and their data frameworks. They should extensively test prospective tools to ensure they satisfy business needs, while simultaneously making sure their employees have the technical skills they’ll need to use these tools.
SEE: Top data quality tools (TechRepublic)
Using such tools and platforms fosters a culture of collaboration by simplifying the movement and replication of employees’ processes, from the business analyst to the data scientist to the automation specialist. These tools help organizations automate mission-critical tasks such as data discovery, data cleansing and transformation, and data monitoring and reporting in particular.